CeCaS – Central Car Server
- Contact:
- Project group:
Prof. Becker
- Funding:
BMBF
- Partner:
28 Partner, u.a. Infineon, BOSCH und TU München
- Startdate:
01.12.2022
- Enddate:
30.11.2025
CeCaS - Central Car Server
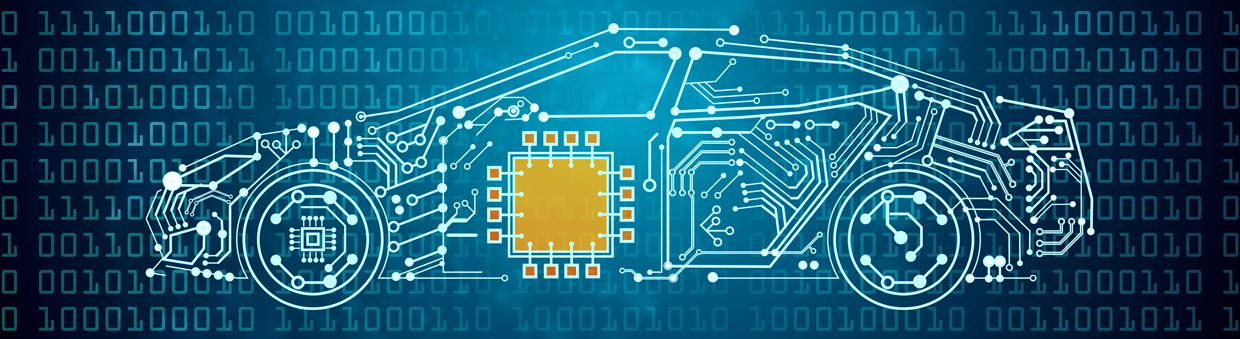
Problem definition
Automated, connected and electrified vehicles are gaining traction. However, to achieve full everyday suitability, there needs to be energy-efficient and cost-effective high-end compute platforms that keep pace with the requirements for computing power and complexity with full automotive qualification (ASIL-D). In particular AI-based subject areas such as autonomous driving require customized, real-time capable and energy-efficient high-performance processors.
It's all about performance and safety from a single source - and the future viability of the automotive industry.
Project goals
CeCaS creates the processor- and SW-side basis for heterogeneous real-time capable high-performance central computers in vehicles. The aim is to create a combination of safety and high performance tailored to the automotive sector as well as processors, interfaces and system architectures designed specifically for the automotive sector. In short: automotive supercomputing.
ITIV participation
One aspect that ITIV is working on is the design of highly effective and reliable multipurpose hardware accelerators for image processing and for AI in the automobile. The accelerators are connected via high-speed interfaces and integrated into the high-performance processors in the Central Car Server.
In order to efficiently distribute the computing load in the system, ITIV is also working on the partitioning of neural networks. By shifting the workload to platforms close to the sensor, the system is optimized with regard to various metrics.
In addition, benchmarks are considered to evaluate hardware accelerators for AI in vehicles to assess the trade-off of accuracy and performance. Integration of the benchmarks into an automated design space exploration will enable the hardware-optimized architecture search for application-specific AI accelerators.