Dipl.-Specialist Iuliia Topko
- Member of Scientific Staff
- Group: Prof. Becker
- Room: 126
CS 30.10 - Phone: +49 721 608-46500
- iuliia topko ∂does-not-exist.kit edu
Engesserstr. 5
76131 Karlsruhe
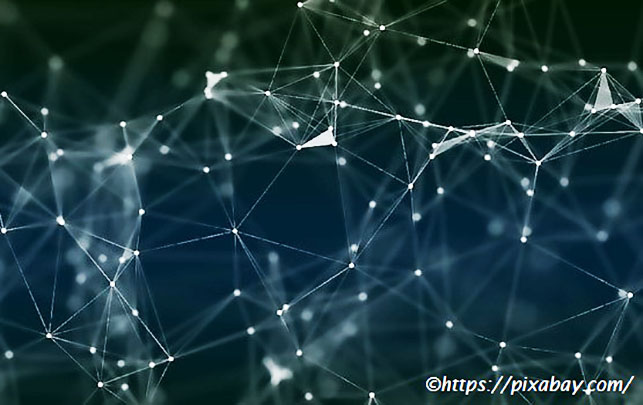
Federated Learning
Federated Learning (FL) is a distributed machine learning paradigm used for decentralized training on a large number of endpoints. Each end-device stores data locally and collaboratively learns a shared predictive model. The security of user data and the unlimited number of devices involved are the main advantages of this approach. FL can be used in healthcare, autonomous driving, and IoT systems where the number of connected devices varies from thousands to millions.
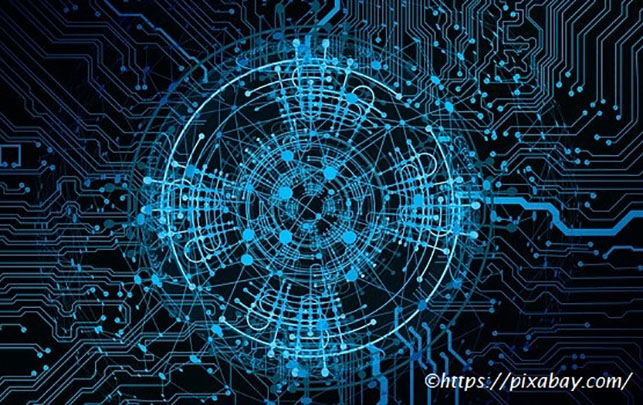
AI Accelerator Architecture
In recent years, artificial intelligence (AI) and machine learning techniques have been used in a variety of technologies such as communications, autonomous driving, and smart industry. Current GPU platforms are not suitable for low-power applications, such as edge applications. To enable faster and more power-efficient processing of AI workloads, a new accelerator architecture is required. FPGA are the most promising hardware platforms due to their flexibility and parallelization capabilities.
Titel | Typ |
---|---|
Implementation of measurement of power efficiency of computing platforms | Bachelorarbeit |
Implementation of a combined metric for the assessment of different platform/DNN experiments | Bachelorarbeit |
On-device training | Masterarbeit |
Publications
Schmidt, P.; Topko, I.; Stammler, M.; Harbaum, T.; Becker, J.; Berner, R.; Ahmed, O.; Jagielski, J.; Seidler, T.; Abel, M.; Kreutzer, M.; Kirschner, M.; Betancourt, V. P.; Sehm, R.; Groth, L.; Neskovic, A.; Meyer, R.; Mulhem, S.; Berekovic, M.; Probst, M.; et al.
2024. 27th Design, Automation and Test in Europe Conference and Exhibition (DATE 2024), 6 S., Institute of Electrical and Electronics Engineers (IEEE). doi:10.23919/DATE58400.2024.10546796
Topko, I.; Harbaum, T.; Becker, J.
2024. 2024 IEEE Nordic Circuits and Systems Conference (NorCAS). Ed.: J. Nurmi, 7 S., Institute of Electrical and Electronics Engineers (IEEE). doi:10.1109/NorCAS64408.2024.10752456
Serdyuk, A.; Kreß, F.; Topko, I.; Harbaum, T.; Becker, J.; Hamann, T.; Kämpf, P.
2024. 2024 Sensor Data Fusion: Trends, Solutions, Applications (SDF), Bonn, Germany, 25-27 November 2024, Institute of Electrical and Electronics Engineers (IEEE). doi:10.1109/SDF63218.2024.10773805
Kreß, F.; Sidorenko, V.; Topko, I.; Unger, K.; Harbaum, T.; Becker, J.
2024. 2024 IEEE 3rd German Education Conference (GECon), Munich, Germany, 05-07 August 2024, Institute of Electrical and Electronics Engineers (IEEE). doi:10.1109/GECon62014.2024.10734007
Harbaum, T.; Topko, I.; Serdyuk, A.; Fürst-Walter, I.; Kreß, F.; Becker, J.
2024. 3rd Workshop on Deep Learning for IoT (DL4IoT-2024)
Kreß, F.; Sidorenko, V.; Topko, I.; Unger, K.; Schneider, M.; Becker, J.
2024
Kreß, F.; Sidorenko, V.; Topko, I.; Unger, K.; Schneider, M.; Becker, J.
2024
Kreß, F.; Sidorenko, V.; Topko, I.; Unger, K.; Schneider, M.; Becker, J.
2024
Kreß, F.; Sidorenko, V.; Topko, I.; Unger, K.; Schneider, M.; Becker, J.
2024
Kreß, F.; Sidorenko, V.; Topko, I.; Unger, K.; Schneider, M.; Becker, J.
2024
Kreß, F.; Sidorenko, V.; Topko, I.; Unger, K.; Schneider, M.; Becker, J.
2024
Kreß, F.; Sidorenko, V.; Topko, I.; Unger, K.; Schneider, M.; Becker, J.
2024
Kreß, F.; Sidorenko, V.; Topko, I.; Unger, K.; Schneider, M.; Becker, J.
2024
Kreß, F.; Sidorenko, V.; Topko, I.; Unger, K.; Schneider, M.; Becker, J.
2024
Topko, B.; Topko, Y.; Khabarov, S.; Zamyatin, N.; Zubarev, E.
2022. IEEE Transactions on Nuclear Science, 69 (1), 98–104. doi:10.1109/TNS.2021.3136944
Topko, Y.; Khabarov, S.; Topko, B.; Kovalev, Y.; Zamyatin, N.; Tarasov, O.; Zubarev, E.
2022. IEEE Transactions on Nuclear Science, 69 (3), 634–638. doi:10.1109/TNS.2022.3150753
Topko, Y.; Topko, B.; Khabarov, S.; Zamyatin, N.
2022. Nuclear Instruments and Methods in Physics Research Section A: Accelerators, Spectrometers, Detectors and Associated Equipment, 1033, Artkl.Nr.: 166680. doi:10.1016/j.nima.2022.166680
BM@N Collaboration; Patsyuk, M.; Kahlbow, J.; Laskaris, G.; Duer, M.; Lenivenko, V.; Segarra, E. P.; Atovullaev, T.; Johansson, G.; Aumann, T.; Corsi, A.; Hen, O.; Kapishin, M.; Panin, V.; Piasetzky, E.; Abraamyan, K.; Afanasiev, S.; Agakishiev, G.; Alekseev, P.; Ivanova, Y.; et al.
2021. Nature Physics, 17 (6), 693–699. doi:10.1038/s41567-021-01193-4